Chain-of-Query Prompting for Efficient Small Language Models in Multi-Hop Open-Domain Question Answering
Dec 15, 2024·,
,,,·
0 min read
Rithika Akula
Roland Oruche

Zian Zeng
Yuanxun Zhang
Marcos Zampieri
Prasad Calyam
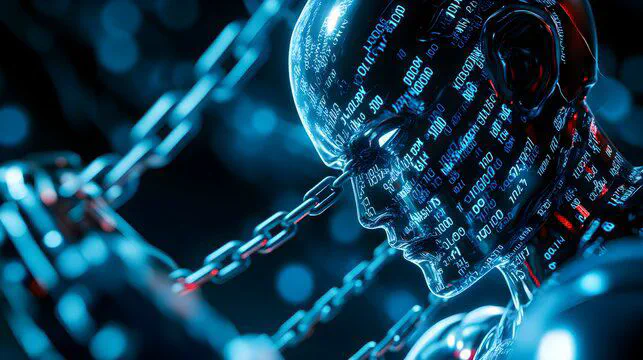
Abstract
Large Language Models (LLMs) have been shown to exhibit robust performance in multi-hop open-domain question- answering (ODQA), which is often attributed to a large number of parameters and various prompting formats. While smaller language models (LMs) offer a more cost-effective approach for real-world applications, these LMs are often challenged with maintaining factual responses in multi-hop ODQA settings. In this paper, we introduce a novel prompting approach viz., Chain-of-Query (CoQ), that is designed to enhance smaller LMs by decomposing complex queries into context-aware subqueries for robust ODQA in multi-hop settings. Our CoQ prompting approach creates an efficient pipeline that integrates a retriever with LMs for optimizing the retrieval process through multiple query generation, thereby adding external knowledge to the LM with a small amount of context. We validate our CoQ approach on benchmark datasets for multi-hop ODQA against large-scale LMs with state-of-the-art prompting formats. When evaluating our CoQ prompting approach for small-scale and large-scale LMs, the results demonstrate a significant increase in QA performance by up to 5.4% and 11.5%, respectively, thus, making it a valuable advancement for complex QA tasks.
Type
Publication
2024 IEEE International Conference on Big Data workshop From Theory to Practice: Workshop on Large Language and Foundation Models